One question lots of mass market investors are asking these days is “where can I invest to participate in artificial intelligence expected growth?”
Ironically, the answers are nearly identical to the answers to the question “where can I invest to participate in internet-related or digital economy growth?”
Unless you own or work for a venture capital firm, or are an accredited investor, you will not be able to invest in startups oriented around artificial intelligence, leaving you with the task of identifying existing public firms that have some plausible direct relationship to AI.
Eventually, as has proven to be true for the internet, most firms will have some indirect relationship to the internet, but that is not so helpful in identifying candidates for investment right now.
As is true for just about any general-purpose technology before it (steam power, railroads, the internet, electricity) and other platforms that might not always be considered GPTs (highway systems, passenger air travel, mobile communications, telephone systems), infrastructure is where investments must be made first, before the full range of use cases develops.
So for “regular people” the domain initially will be public firms with AI infrastructure operations: the compute power to run AI software, the products required to build AI models, make inferences, create applications or supply platforms and devices to run the models and make inferences, sustain the connectivity to processing nodes, create and run the data centers, provide AI functions as a service.
Most of these infra segments includes firms one might own for other reasons as well (dividends, revenue growth, capital appreciation, real estate investments, software or information technology, content assets, connectivity).
So, perhaps oddly enough, “AI investments” are pretty much the same as would be expected if one were instead searching for “digital economy” or “internet” investments, with perhaps a stronger weighting towards “picks and shovels” that create or sustain the infrastructure to run apps and provide experiences.
Analyst Cody Willard suggests an 11-layer model focused on AI infrastructure, including some private firms or open-source initiatives, but also focusing on public firms plausibly involved in creating AI infrastructure, applications and content.
Chips, servers, data centers, cloud computing, data management, algorithms, models, internet connectivity and end user devices are perhaps the clearest examples of AI infra. But some might also include content, enterprise AI-enabled applications or advertising as layers of the AI value chain.
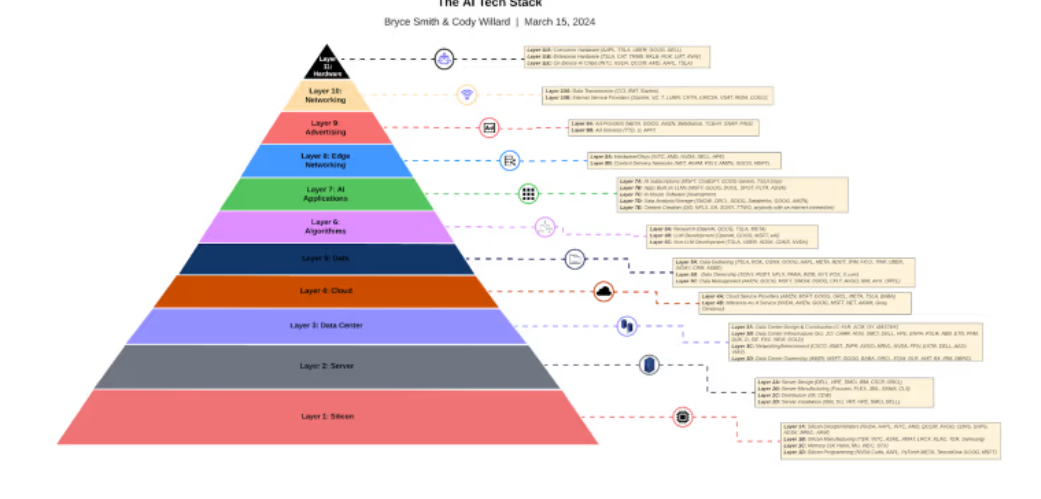
source: Cody Willard, MarketWatch
Looking at infra as 11 or more layers, that might suggest a layer one (chips) including
Silicon (Nvidia, duh!), Intel, AMD, Qualcomm and Broadcom (add Microsoft, Alphabet, Meta and Apple to the extent they are developing their own AI chips as well)
Silicon design services such as Cadence Design Systems, Synopsis or Autodesk
Application Specific Integrated Circuit (ASIC) designer such as Broadcom , Marvel, Intel, Advanced Micro Devices and Qualcomm
Silicon intellectual property including Arm, Intel
Semiconductor equipment such as ASML, Advanced Materials, Lam Research, KLA Corp. and Teradyne
Foundries including TSMC, Intel, Samsung, Global Foundries
Memory (SK Hynix, Samsung, Micron, Western Digital, Seagate
Machine Learning languages including PyTorch (open-sourced from Meta), TensorFlow (open-sourced from Google), Keras, Microsoft Cognitive Toolkit, Theano, Apache MXNet, Chainer, JAX, TensorFlow.js
Layer two might focus on servers, including:
Server design (Dell, Hewlett-Packard Enterprises, Super Micro, IBM, Lenovo, Cisco, Oracle, Fujitsu, Quanta Cloud Technology, Inspur
Server manufacturing including Foxconn, Flex, Jabil, Sanmina Corp., Pegatron Corp., Celestica, Wistron Corp., , Quanta Computer,, Compal Electronics, Inventec Corp..
Distribution partners include Ingram Micro, Aero Electronics and CDW.
Server installation services (IBM, Schneider Electric, Vertiv Holdings Co., Hewlett Packard Enterprise, Super Micro, Dell
Layer three can be viewed as data centers:
Data center design and construction (Holder Construction, Turner Construction, Jacobs, Fluor Corporation, AECOM, Syska Hennessy Group, Corgan, Gensler, HDR, Mastek, Dycom
Data center Infra, especially cooling (Schneider Electric, Johnson Controls, Carrier Global Corporation, Honeywell International Inc., Siemens AG, Super Micro, Dell, Hewlett-Packard Enterprises
Electric components, including renewable energy, including Enphase Energy, Inc., Solaredge, First Solar, Tesla, Inc., SunPower Corporation, Schneider Electric, ABB Ltd., Eaton Corporation
Electrical power suppliers (PNM Resources, NextEra Energy, Duke Energy Corporation, Dominion Energy), Southern Company, Exelon Corporation, American Electric Power Company, PG&E Corp., Consolidated Edison, Xcel Energy, Entergy Corp.
Electric utility infra (General Electric, Siemens Energy, Mitsubishi Heavy Industries, Toshiba Corporation, Hitachi Ltd., ABB Ltd., BWX Technologies, Doosan Heavy Industries & Construction
Raw materials such as copper, gold, plastic (oil) and silver (Freeport-McMoran, Newmont Corp., Barrick Gold Corp., Franco-Nevada Corp., Freeport-McMoRan Inc., Southern Copper Corporation, BHP Group, ExxonMobil, Chevron, ConocoPhillips
Networking and interconnect gear (Cisco Systems, Arista Networks, Inc., Juniper Networks, Inc., Broadcom Inc., NVIDIA Corp.), F5 Networks, Extreme Networks, Inc., Dell Technologies, Marvel, Applied Optoelectronics, Viavi Solutions, MaxLinear, Emcore, Nlight
Layer four might be envisioned as the “cloud” layer, including:
Cloud data centers (Amazon Web Services, Microsoft Azure, Google Cloud Platform, Alibaba, Oracle, Tesla, Meta
Data center real estate investment trusts (Equinix, Digital Realty Trust, Inc., CyrusOne Inc. (KKR), CoreSite Realty Corporation (subsidiary of American Tower), QTS Realty Trust (Blackstone)), Iron Mountain Inc., DigitalBridge
Cloud computing as a service providers (AWS, Microsoft Azure, GCP, Oracle, Meta, Tesla, Alibaba
Inference As A Service (NVIDIA, Amazon Web Services, Google Cloud AI Platform, Microsoft, Cloudflare, Akamai
Layer five might be viewed as the data layer, including functions such as data gathering and input, machine vision, data sources:
Machine vision including Tesla, Rockwell Automation, Zebra, Cognex Corp., Keyence Corp., OMRON Corp., Basler AG, Teledyne Technologies, ISRA VISION AG
Consumer data sources (shopping, other behavior including Meta, ByteDance, Google, Apple, Amazon, Snap, Pinterest, Yelp, Tencent, Reddit, Etsy,, Wayfair, Walmart
Financial data (Apple Pay, Google Pay, JPMorgan Chase & Co., Visa Inc., Mastercard Inc., Discover Financial Services, PayPal Holdings, Inc., Square, Inc., Robinhood Markets, Inc., Morgan Stanley, The Charles Schwab Corp., Fair Isaac Corp., TransUnion, Equifax Inc.
Location, travel data (Apple Inc., Alphabet Inc., Verizon Communications Inc., AT&T Inc., T-Mobile US, Inc., Uber Technologies, Inc., Lyft, Inc., Expedia, Tripadvisor
Enterprise Data (ServiceNow, Apple, Salesforce.com, Inc., Oracle Corp., Microsoft Corp., Alphabet Inc., Dropbox, Inc., Box, Inc., Workday, Inc., AutoDesk, Adobe, Dassault Systèmes, PTC Inc., Ansys, Inc., Trimble Inc., Siemens AG, AVEVA Group plc, Bentley Systems, Inc..
Content (Disney, Sony, Spotify, Netflix, Warner Brothers, Paramount, New York Times, Fox, Simon & Shuster, Random House
Data Management (Amazon Web Services, Alphabet Inc., Microsoft Corp., Snowflake Inc, Datadog, MongoDB, Inc., Oracle Corp., Confluent, Inc., Broadcom Inc., Alteryx, Inc., International Business Machines Corp., Cisco Systems, Inc.
Layer six is the algorithm and model layer:
Algorithms (OpenAI, Google Deepmind, Tesla AI, Meta Labs
Large language models (OpenAI, Google, Microsoft, Anthropic, Perplexity) and training
Libraries: Hugging Face
Layer seven is the applications layer:
Generative AI as an app (ChatGPT, Gemini, Anthropic, xAI Grok, LLaMA, Stability AI, Mistral, Mosaic, Amazon
Apps using LLM (Microsoft Copilot & Github, Office365, Google Workspace, Duolingo, Alexa, Siri, Spotify, Palantir, Autodesk, Unity
Data analysis (Snowflake, Oracle, Datadog, AWS, Google, Azure
Content Creation (The Walt Disney Company, Netflix, Inc., Electronic Arts Inc., Warner Bros. Discovery, Inc., Paramount Global, Sony Group Corp. , Comcast Corp. , Activision Blizzard, Inc. (Microsoft), Electronic Arts, Take-Two Interactive Software, Inc., Spotify Technology S.A., Lions Gate Entertainment Corp, Fox
Cybersecurity (Palo Alto Networks, Fortinet, , CrowdStrike Holdings,, Zscaler, , Check Point Software Technologies Ltd. , CyberArk Software Ltd., Okta, Inc., FireEye, Inc.
Layer eight might be edge networking:
Hardware and servers (Intel, AMD, NVIDIA, Dell, HPE)
Content Delivery Networks (Cloudflare, Akamai, Fastly, AWS, GCP, Azure)
Layer nine might be advertising:
Venues (Meta, Google, Amazon, Snapchat, Pinterest)
Ad placement and services (The Trade Desk, Unity, Applovin)
Layer 10 might be networking:
Tower infra (Crown Castle International Corp., American Tower, SBAC)
Access providers (Starlink, Verizon Communications, AT&T, Lumen Technologies, Charter Communications, T-Mobile, Comcast, Comtech, ViaSat, Iridium Communications, Gogo
Layer 11 includes end user devices:
Consumer devices including Apple iPhone, iPad, Mac, PCs, Google Pixel phones, Meta Glasses, Lenovo PCs
Enterprise devices such as robots (Tesla Optimus, Mitsubishi, Kawasaki, Epson, Universal Robots, Omron, Yaskawa Electric, Fanuc, Kuka, Denso, ABB
AI machinery (tractors, cranes, containers, and boats made by Caterpillar, John Deere, Trimble)
AI satellites and spacecraft: SpaceX, Rocket Lab, Intuitive Machines, Optimus by Tesla)
AI Drones by AeroVironment, Lockheed Martin, Boeing, Northrop Grumman
Some of us might argue that mass market investors should view virtually all these assets as categories we’d consider for other reasons, despite their AI potential, as it might be some time before AI revenues are material.
In Willard’s 11-layer model, some of us might consider much of layer seven and virtually all of layers eight through 11 as being part of the broader computing and internet value chains, and not specifically powered by AI potential.
And parts of layers one through six would be required to support modern computing, even if AI did not exist. The point is that the AI value chain overlaps substantially with the internet value chain. With a few specific exceptions, Nvidia being the primary example, virtually all the other assets in the developing AI value chain would also be candidates for ownership as part of the internet value chain.