By some estimates generative artificial intelligence infrastructure investments are 10 times the revenue currently generated by those investments.
Perhaps the good news is that costs of deriving inferences (using generative AI) appear to be dropping sharply, and value could be surfacing.
Generative artificial intelligence reduces document parsing times for Flexport, a logistics company that has to process shipping contracts and bills of lading, reducing the time spent by as much as 80 percent, according to the firm’s engineering director. That matters as end user firms will have to justify their spending on GenAI.
As expensive as generative artificial intelligence models have been to create, train and use to derive inferences, costs are coming down, as one would expect for any digital technology. Inference costs, for example, have dropped dramatically over the last year, according to the State of AI Report 2024.

source: State of AI 2024
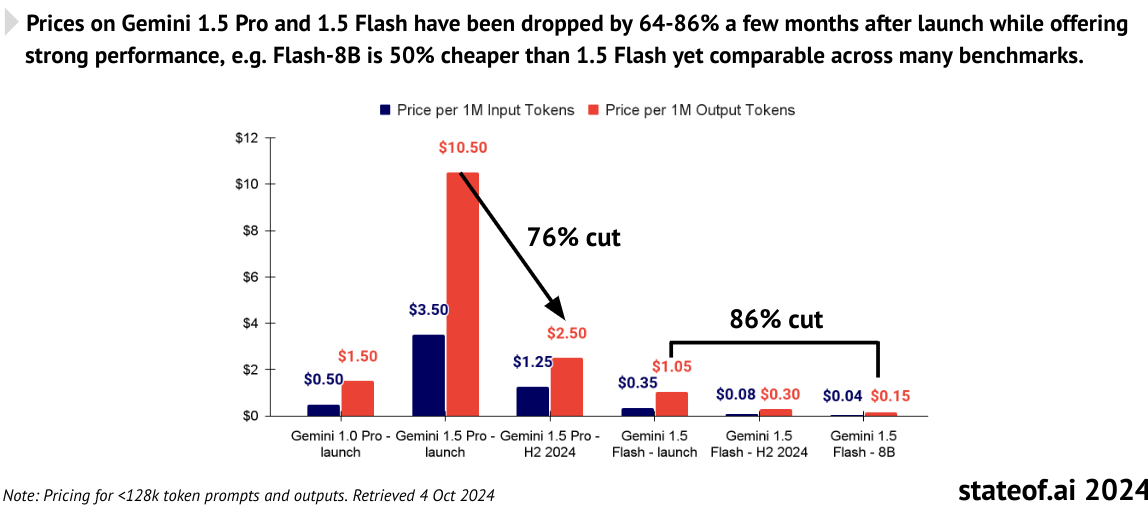
source: State of AI 2024
Up to this point, it has generally also been true that model accuracy also has been directly related to model size, while model size is directly related to infrastructure (compute capability) cost.
But developers seem to be discovering that output can be achieved using smaller models, which should, in turn, reduce the cost of creating models.
Study/Report | Date | Publisher | Key Conclusion |
Mistral 7B release | 2023 | Mistral AI | Mistral 7B outperforms Llama 2 13B on most benchmarks despite being almost half the size, demonstrating the effectiveness of smaller, more efficient models1. |
Phi-2 release | 2023 | Microsoft | The 2.7B parameter Phi-2 model matches or outperforms larger models like GPT-3.5 on various benchmarks, showcasing the potential of smaller, well-trained models2. |
TinyLlama release | 2024 | TinyLlama Team | TinyLlama, a 1.1B parameter model, achieves performance comparable to Llama 2 7B on certain tasks, highlighting the efficiency of compact models3. |
Gemma release | 2024 | Google | Gemma 2B and 7B models demonstrate strong performance relative to their size, competing with larger open-source models in various benchmarks4. |
RMKV-x060 release | 2024 | RMKV Team | The model, with only 1.6B parameters, shows competitive performance against much larger models, emphasizing the potential of efficient architectures5. |
Smaller models also mean it is possible to run GenAI on edge devices, rather than having to process data remotely, which opens up new possibilities for use cases, including voice interaction; language translation; image recognition; device anomaly detection; transportation and security, for example.
Any use case requiring low latency, low energy consumption, lower processing cost or higher security might benefit from on-board edge processing.
Training costs also have been declining since 2020.
Study/Report Name | Date | Publishing Venue | Key Conclusion |
GPT-4o mini release | 2024 | OpenAI | GPT-4o mini offers a 60% cost reduction compared to ChatGPT 3.5 Turbo, making generative AI more affordable for developers. |
Llama 3.1 release | 2024 | Meta | Llama 3.1 provides open-source language models rivaling proprietary ones, offering a cost-effective alternative for businesses. |
Mistral Large 2 release | 2024 | Mistral AI | Mistral Large 2 offers a more powerful open-source model, providing another cost-effective option for generative AI implementation. |
AI Cost Savings Report | 2024 | Virtasant | While current operational costs for generative AI are high, true cost savings are expected to emerge as companies optimize usage and technology improves. |
Generative AI 2023 Report | 2023 | AI Accelerator Institute | Although cost savings weren't a primary driver of generative AI adoption (only 1.2% of respondents), efficiency gains (26.7%) suggest potential for indirect cost reductions. |
And it might be fair to note that much of the AI infra investment is heavy because it requires expensive servers and other physical assets. It's more akin--on one hand--to building roads, dams, bridges and airports than writing code to create software applications. The payback periods therefore will be longer.
On the other hand, AI infra investments also are akin to venture capital: high stakes investments in uncertain ventures.
The concern some seem to have is that there will not be a payback at all, or a near-term payback. Such questions cannot be definitively answered at the moment.
What does seem more likely is that, eventually, at least a few big winners will be produced. So many of the investments might mimic venture capital returns overall: a few big winners; some breakeven bets and some that actually lose money.
No comments:
Post a Comment